LÍNEAS DE INVESTIGACIÓN
En nuestro equipo de investigación la curiosidad nunca se agota. Cada uno de nosotros(as) aporta una perspectiva única, una habilidad especial y pasión a este equipo. Nos complementamos y nos desafiamos constantemente para alcanzar nuevas alturas. No importa cuánto hayamos logrado hasta ahora, siempre sabemos que hay más por descubrir, explorar y crear. La IA es un campo en constante evolución, y estamos aquí para liderar el camino.
RL1 / Aprendizaje profundo para visión y lenguaje
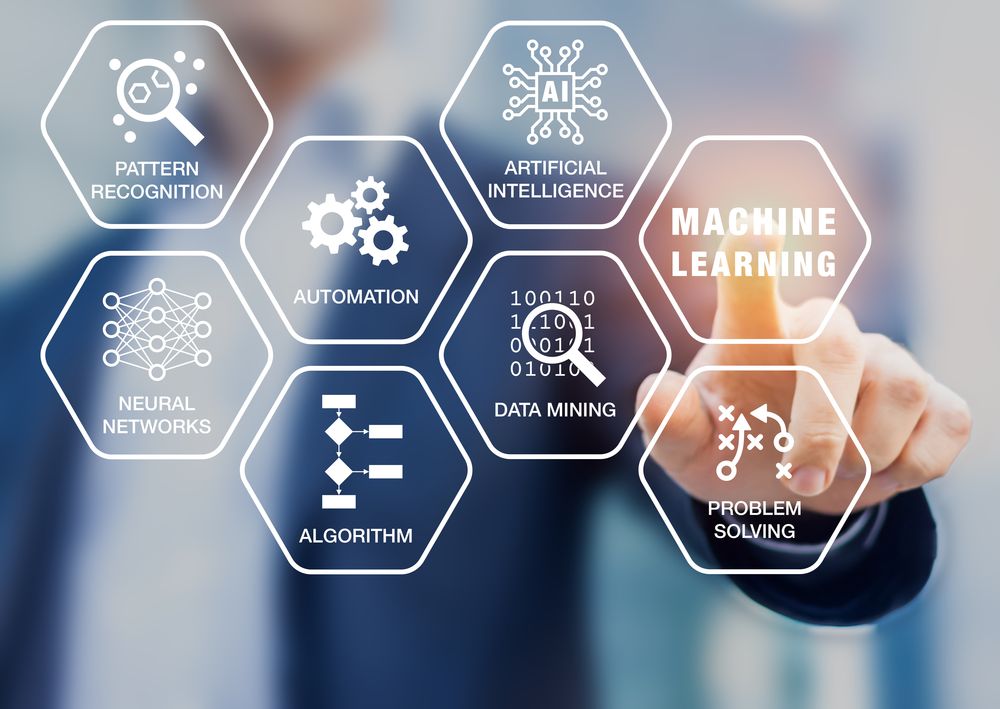
Nuevas teorías y métodos para continuar desentrañando el potencial del Aprendizaje Profundo para crear sistemas cognitivos avanzados con un enfoque en la visión y el lenguaje
RL2 / IA neuro-simbólica
Integración de la IA lógica-probabilística y la basada en el aprendizaje profundo, invocando mutuamente las soluciones de cada parte, inyectando y utilizando la semántica en el aprendizaje profundo.
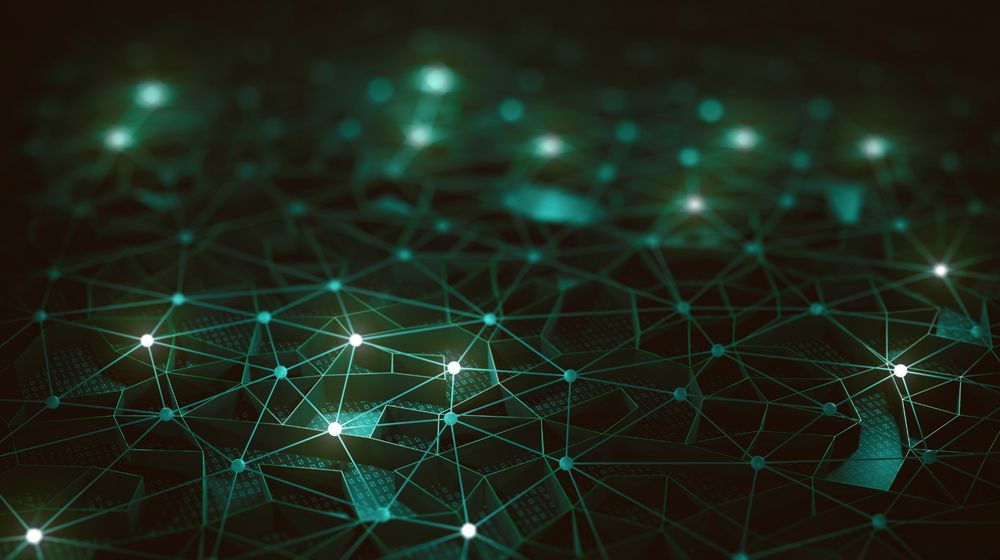
RL3 / IA inspirada en el cerebro
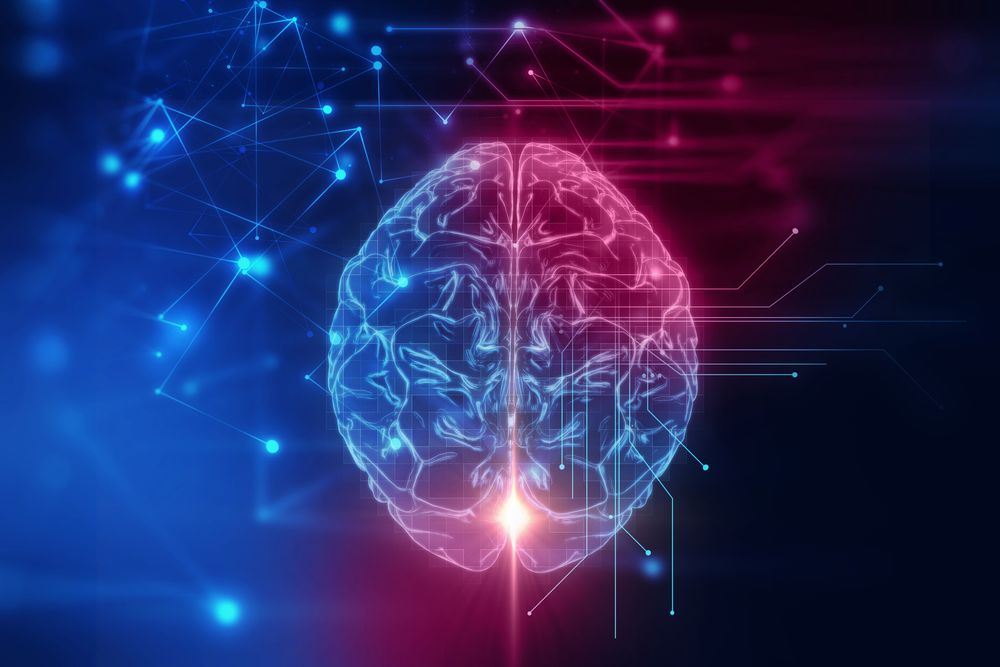
Reunir a científicos de la neurociencia, la psicología cognitiva y la IA para explotar los conocimientos de las operaciones anatómicas y cognitivas de los cerebros biológicos para iluminar a los investigadores de la IA.
RL4 / Aprendizaje automático basado en la física
Reunir a matemáticos, físicos y científicos de la IA para explotar los conocimientos de las ciencias físicas para desarrollar modelos de aprendizaje de máquina basados en relaciones causales.
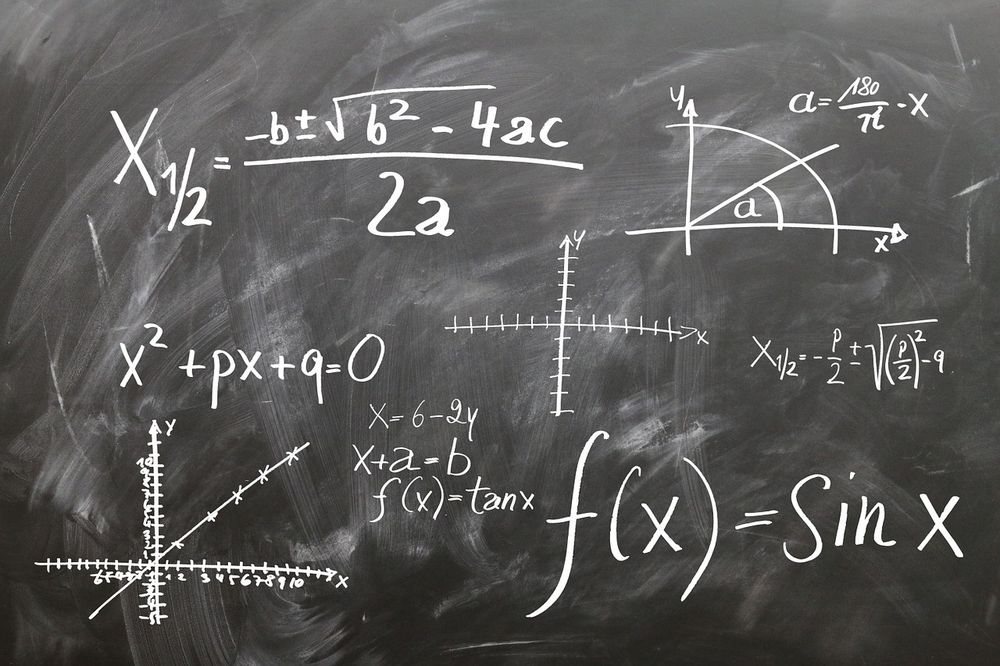
RL5 / IA centrada en las personas
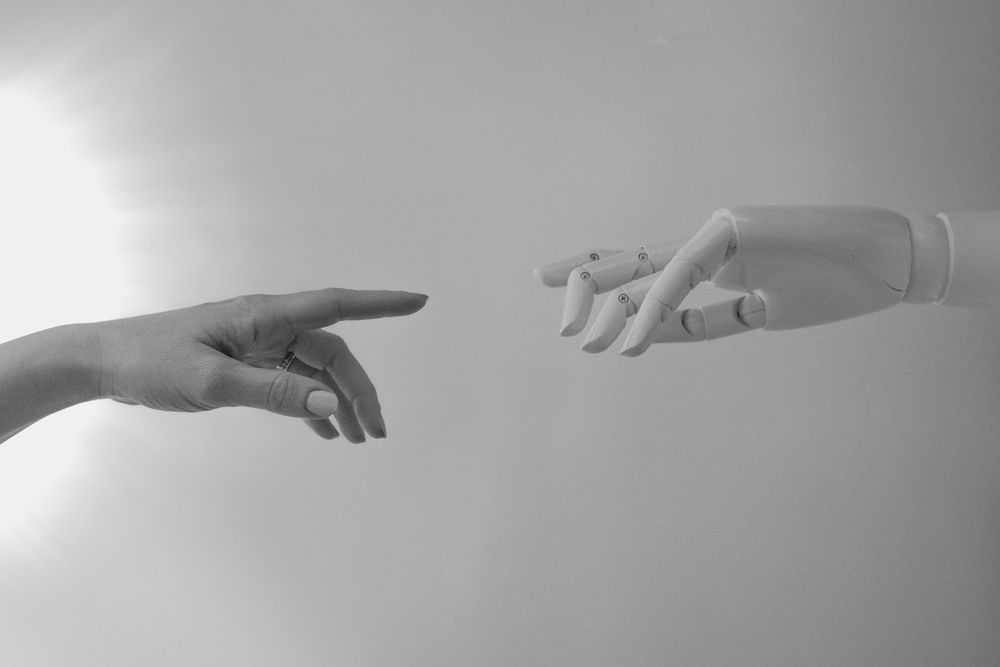
Nuevas tecnologías para un uso justo, seguro y transparente de la IA en la sociedad, así como metodologías para evaluar su impacto en la misma. Promover nuevas herramientas para una IA interpretable y explicable.
Investigadores(as) principales
Investigadores(as) Cenia
La meta de este grupo de investigadores(as) permanentes es producir investigación de frontera en IA, a través de colaboraciones internas, con las otras líneas de investigación, nacionales e internacionales. Reunimos a expertos(as) en visión, procesamiento del lenguaje natural, integración multimodal, aprendizaje adaptativo, redes de grafos y neurociencia. También participan con el equipo de transferencia tecnológica para desarrollar aplicaciones de IA en smart cities, sustentabilidad, análisis, agricultura con datos satelitales, detección y prevención de incendios, y salud.
Comité Científico Cenia
Investigadores(as) asociados
Investigadores(as) jóvenes
Postdoctorados
Colaboradores(as) asociados
Colaboradores internacionales
ABSTRACT
Continuous learning occurs naturally in human beings. However, Deep Learning methods suffer from a problem known as Catastrophic Forgetting (CF) that consists of a model drastically decreasing its performance on previously learned tasks when it is sequentially trained on new tasks. This situation, known as task interference, occurs when a network modifies relevant weight values as it learns a new task. In this work, we propose two main strategies to face the problem of task interference in convolutional neural networks. First, we use a sparse coding technique to adaptively allocate model capacity to different tasks avoiding interference between them. Specifically, we use a strategy based on group sparse regularization to specialize groups of parameters to learn each task. Afterward, by adding binary masks, we can freeze these groups of parameters, using the rest of the network to learn new tasks. Second, we use a meta learning technique to foster knowledge transfer among tasks, encouraging weight reusability instead of overwriting. Specifically, we use an optimization strategy based on episodic training to foster learning weights that are expected to be useful to solve future tasks. Together, these two strategies help us to avoid interference by preserving compatibility with previous and future weight values. Using this approach, we achieve state-of-the-art results on popular benchmarks used to test techniques to avoid CF. In particular, we conduct an ablation study to identify the contribution of each component of the proposed method, demonstrating its ability to avoid retroactive interference with previous tasks and to promote knowledge transfer to future tasks.
ABSTRACT
Every year physicians face an increasing demand of image-based diagnosis from patients, a problem that can be addressed with recent artificial intelligence methods. In this context, we survey works in the area of automatic report generation from medical images, with emphasis on methods using deep neural networks, with respect to: (1) Datasets, (2) Architecture Design, (3) Explainability and (4) Evaluation Metrics. Our survey identifies interesting developments, but also remaining challenges. Among them, the current evaluation of generated reports is especially weak, since it mostly relies on traditional Natural Language Processing (NLP) metrics, which do not accurately capture medical correctness.
ABSTRACT
This article describes the participation and results of the PUC Chile team in the Turberculosis task in the context of ImageCLEFmedical challenge 2021. We were ranked 7th based on the kappa metric and 4th in terms of accuracy. We describe three approaches we tried in order to address the task. Our best approach used 2D images visually encoded with a DenseNet neural network, which representations were concatenated to finally output the classification with a softmax layer. We describe in detail this and other two approaches, and we conclude by discussing some ideas for future work.
Publisher: Logical Methods in Computer Science, Link>
ABSTRACT
We investigate the application of the Shapley value to quantifying the contribution of a tuple to a query answer. The Shapley value is a widely known numerical measure in cooperative game theory and in many applications of game theory for assessing the contribution of a player to a coalition game. It has been established already in the 1950s, and is theoretically justified by being the very single wealth-distribution measure that satisfies some natural axioms. While this value has been investigated in several areas, it received little attention in data management. We study this measure in the context of conjunctive and aggregate queries by defining corresponding coalition games. We provide algorithmic and complexity-theoretic results on the computation of Shapley-based contributions to query answers; and for the hard cases we present approximation algorithms.
ABSTRACT
Word embeddings are vital descriptors of words in unigram representations of documents for many tasks in natural language processing and information retrieval. The representation of queries has been one of the most critical challenges in this area because it consists of a few terms and has little descriptive capacity. Strategies such as average word embeddings can enrich the queries’ descriptive capacity since they favor the identification of related terms from the continuous vector representations that characterize these approaches. We propose a data-driven strategy to combine word embeddings. We use Idf combinations of embeddings to represent queries, showing that these representations outperform the average word embeddings recently proposed in the literature. Experimental results on benchmark data show that our proposal performs well, suggesting that data-driven combinations of word embeddings are a promising line of research in ad-hoc information retrieval.
ABSTRACT
We present a study of an artificial neural architecture that predict human ocular scanpaths while they are free-viewing different images types. This analysis is made by comparing different metrics that encompass scanpath patterns, these metrics aim to measure spatial and temporal errors; such as the MSE, ScanMatch, cross-correlogram peaks, and MultiMatch. Our methodology begin by choosing one architecture and training different parametric models per subject and image type, this allows to adjust the models to each person and a given set of images. We find out that there is a clear difference in prediction when people free-view images with high visual content (high-frequency contents) and low visual content (no-frequency contents). The input features selected for predicting the scanpath are saliency maps calculated from foveated images together with the past of the ocular scanpath of subjects, modeled by our architecture called FovSOS-FSD (Foveated Saliency and Ocular Scanpath with Feature Selection and Direct Prediction).
The results of this study could be used to improve the design of gaze-controlled interfaces, virtual reality, as well as to better understand how humans visually explore their surroundings and pave a way to make future research.
ABSTRACT
ABSTRACT
Background
We examine the efficacy of an online collaborative problem-solving (CPS) teaching approach in academic performance and student connections with other peers, among first-year engineering calculus students at a Latin American university. Our research uses communities of practice (CoP) to emphasize the social nature of learning and the importance of participation and interaction within a community.Methods
The work applies a quasi-experimental design and social network analysis (SNA). A total of 202 engineering students were instructed using CPS methodology (experimental group), while 380 students received traditional online teaching methods (control group) during one semester in the first calculus class for engineers.Results
Results show no significant difference in the grades obtained between the experimental and control groups. However, students exposed to CPS reported a statistically significant higher passing rate, as well as larger and more significant academic and social connections. Additionally, SNA results suggest that CPS facilitated stronger peer connections and promoted a more equitable distribution of participation among students, particularly women, compared to students taught under traditional online teaching methods.Conclusions
The study underscores the importance of fostering collaborative learning environments and highlights CPS as a strategy to enhance student performance and network formation. Findings suggest that CPS can improve academic outcomes and promote more equitable learning practices, potentially reducing dropout rates among women engineering students. These findings contribute to the ongoing efforts to address systematic biases and enhance learning experiences in engineering education.Embeddings are core components of modern model-based Collaborative Filtering (CF) methods, such as Matrix Factorization (MF) and Deep Learning variations. In essence, embeddings are mappings of the original sparse representation of categorical features (eg, user and items) to dense low-dimensional representations. A well-known limitation of such methods is that the learned embeddings are opaque and hard to explain to the users. On the other hand, a key feature of simpler KNN-based CF models (aka user/item-based CF) is that they naturally yield similarity-based explanations, ie, similar users/items as evidence to support model recommendations. Unlike related works that try to attribute explicit meaning (via metadata) to the learned embeddings, in this paper, we propose to equip the learned embeddings of MF with meaningful similarity-based explanations. First, we show that the learned user/item …